matplotlib exercises#
import matplotlib.pyplot as plt
import numpy as np
Q1: planetary positions#
The distances of the planets from the Sun (technically, their semi-major axes) are:
a = np.array([0.39, 0.72, 1.00, 1.52, 5.20, 9.54, 19.22, 30.06, 39.48])
These are in units where the Earth-Sun distance is 1 (astronomical units).
The corresponding periods of their orbits (how long they take to go once around the Sun) are, in years
P = np.array([0.24, 0.62, 1.00, 1.88, 11.86, 29.46, 84.01, 164.8, 248.09])
Finally, the names of the planets corresponding to these are:
names = ["Mercury", "Venus", "Earth", "Mars", "Jupiter", "Saturn",
"Uranus", "Neptune", "Pluto"]
(technically, pluto isn’t a planet anymore, but we still love it :)
Plot as points, the periods vs. distances for each planet on a log-log plot.
Write the name of the planet next to the point for that planet on the plot
Q2: drawing a circle#
For an angle \(\theta\) in the range \(\theta \in [0, 2\pi]\), the polar equations of a circle of radius \(R\) are:
We want to draw a circle.
Create an array to hold the theta values—the more we use, the smoother the circle will be
Create
x
andy
arrays fromtheta
for your choice of \(R\)Plot
y
vs.x
Now, look up the matplotlib fill()
function, and draw a circle filled in with a solid color.
Q3: Circles, circles, circles…#
Generalize your circle drawing commands to produce a function,
draw_circle(x0, y0, R, color)
that draws the circle. Here, (x0, y0)
is the center of the circle, R
is the radius, and color
is the color of the circle.
Now randomly draw 10 circles at different locations, with random radii, and random colors on the same plot.
Q4: Climate#
Download the data file of global surface air temperature averages from here: https://raw.githubusercontent.com/sbu-python-summer/python-tutorial/master/day-4/nasa-giss.txt
(this data comes from: https://data.giss.nasa.gov/gistemp/graphs/)
There are 3 columns here: the year, the temperature change, and a smoothed representation of the temperature change.
Read in this data using
np.loadtxt()
.Plot as a line the smoothed representation of the temperature changes.
Plot as points the temperature change (no smoothing). Color the points blue if they are < 0 and color them red if they are >= 0
You might find the NumPy where()
function useful.
Q5: subplots#
matplotlib has a number of ways to create multiple axes in a figure – look at plt.subplots()
(http://matplotlib.org/api/pyplot_api.html#matplotlib.pyplot.subplot)
Create an x
array using NumPy with a number of points, spanning from \([0, 2\pi]\).
Create 3 axes vertically, and do the following:
Define a new numpy array
f
initialized to a function of your choice.Plot f in the top axes
Compute a numerical derivative of
f
, $\( f' = \frac{f_{i+1} - f_i}{\Delta x}\)$ and plot this in the middle axesDo this again, this time on \(f'\) to compute the second derivative and plot that in the bottom axes
Q6: Mandelbrot set#
The Mandelbrot set is defined such that \(z_{k+1} = z_k^2 + c\) remains bounded, which is usually taken as \(|z_{k+1}| \le 2\) where \(c\) is a complex number and we start with \(z_0 = 0\)
We want to consider a range of \(c\), as complex numbers \(c = x + iy\), where \(-2 < x < 2\) and \(-2 < y < 2\).
For each \(c\), identify its position on a Cartesian grid as \((x,y)\) and assign a value \(N\) that is the number of iterations, \(k\), required for \(|z_{k+1}|\) to become greater than \(2\).
The plot of this function is called the Mandelbrot set.
Here’s a simple implementation that just does a fixed number of iterations and then colors points in Z depending on whether they satisfy \(|z| \le 2\).
Your task is to extend this to record the number of iterations it takes for each point in the Z-plane to violate that constraint, and then plot that data – it will show more structure
N = 256
x = np.linspace(-2, 2, N)
y = np.linspace(-2, 2, N)
xv, yv = np.meshgrid(x, y, indexing="ij")
c = xv + 1j*y
z = np.zeros((N, N), dtype=np.complex128)
for i in range(10):
z = z**2 + c
m = np.ones((N, N))
m[np.abs(z) <= 2] = 0.0
fig, ax = plt.subplots()
ax.imshow(m)
<matplotlib.image.AxesImage at 0x7f40c08b4cd0>
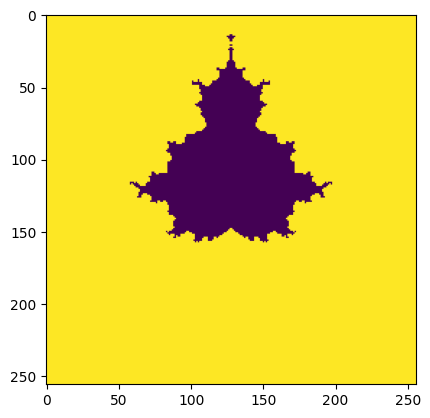